Tracking Exposed
* * *
A collective project that aims to unveil the algorithm opacity
(tracking.exposed)
(Paper: WeTest YouTube )
(Paper: PornHub's Heteronormativity )
Heteronormativity and Pornography
an Algorithmic Analysis of Pornhub
* * *
Tracking Exposed & UniMi
(
Porn Studies
)
we analyze platforms
* * *
NOT PEOPLE
TREX browser extension collects data on .json and .csv formats, in order to decipher the functioning of the proprietary algorithms for public interest.
.csv structure
THE DATASET
* * *
Each entry represent a suggested video from Pornhub.
Each video snippet you might click on while visiting the platform.
{ "title": "Sunny Sextape on the Sofa! Squirt, deepthroat", "authorName": "Leolulu", "authorLink": "/pornstar/leolulu", "duration": "17:15", "href": "/view_video.php?viewkey=ph5e18b11299830", "savingTime": "2020-01-19T22:18:10.522Z", "metadataId": "738c411c67c7b6107bbb3ff8631070011a814f48", "clientTime": "2020-01-19T22:17:48.000Z", "size": 421227, "randomUUID": "INITucmr5condtj2zkfy9o6cv4", "selector": "body", "incremental": 0, "amountGrossDimension": 0, "packet": 0, "type": "home", "processed": true, "step": 0, "session": 1, "pseudo": "blueberry-cake-pistachio", "sectionName": "Hot Porn Videos In United States", "sectionHref": "/video?o=ht&cc=us", "sectionOrder": 0, "displayOrder": 0, },
Our BOTS from guardoni.js helped us
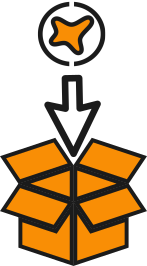
Adding the poTREX extension
By default on every new clean browser profile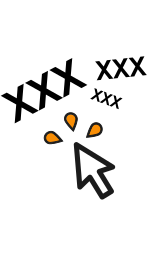
Open homepages
avoiding PTSD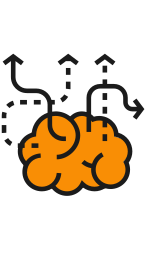
Unravel the algorithmic mist
starting from a .csv releaseTo consider how the platform might reiterate a heteronormative point of view, we created several profiles with the intention to investigate differences in recommended and personalized content.
* * *
to the user accounts were assigned a variable gender identity and a fixed sexual interest during the registration phase
How platforms conceptualise gender has broader effects, as it reifies a specific, socially embedded cultural conception that is able to shape, affect, and maintain gender identities.
What Pornhub looks like ;)
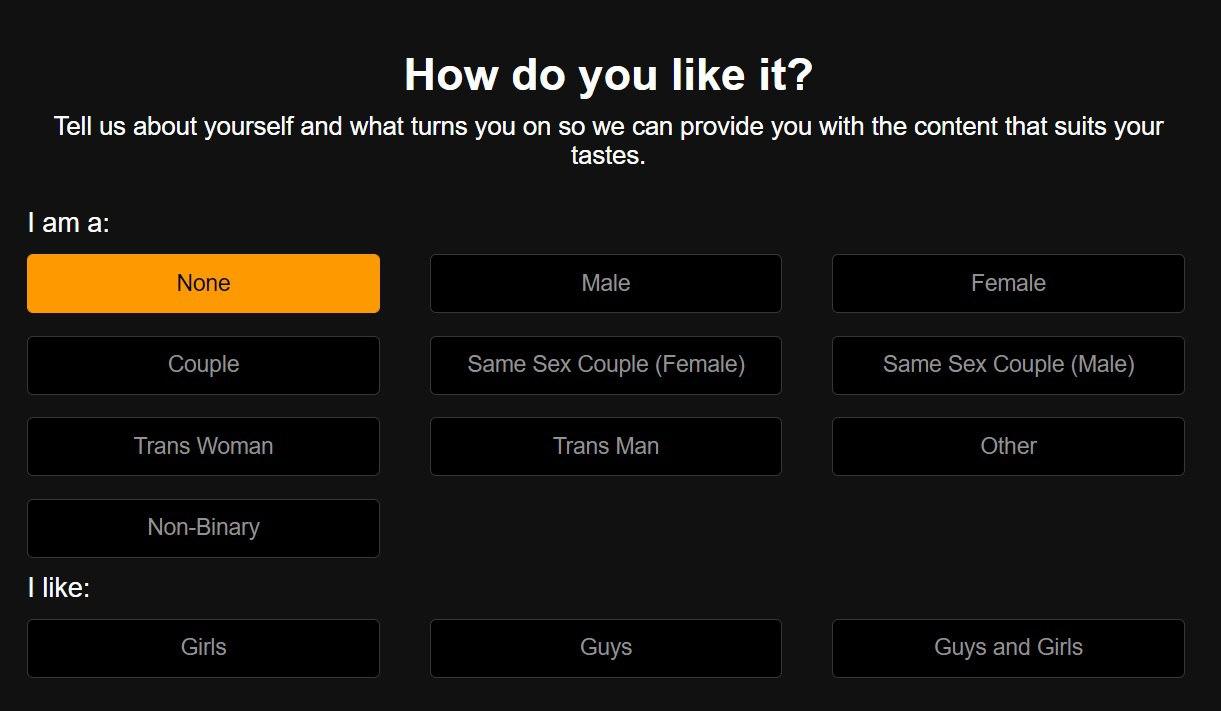
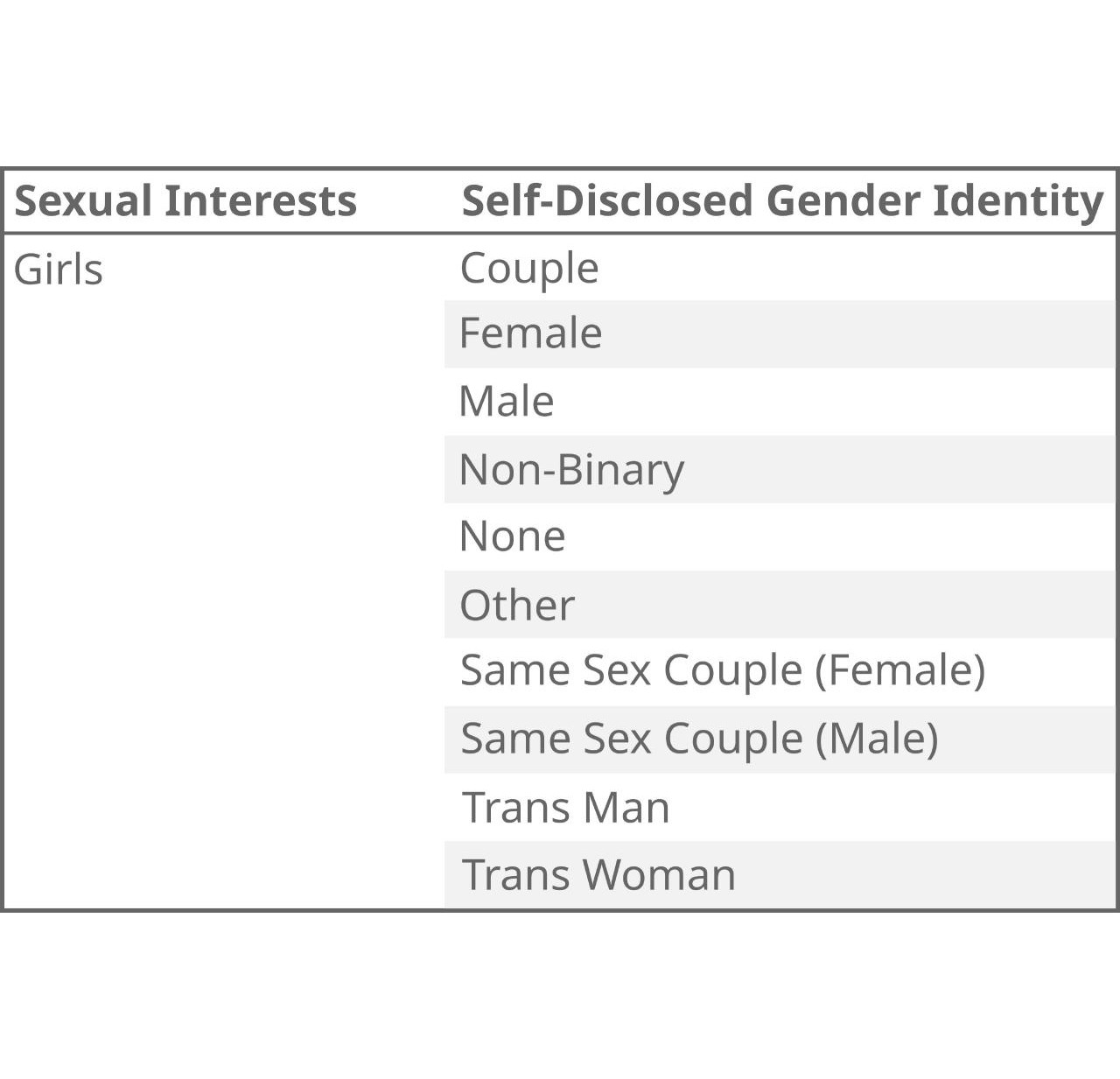
Methodology
‘gender’ and ‘sexual orientation’ are defined by the platform
- Videos per homepage: 46
- Homepages: 1600
- Videos: 45.959
- Reliability: 99.1%
- Unique videos: 118
Data collection processes leveraged on the ‘Pornhub Tracking Exposed’ (poTREX) infrastructure, that collects and processes data from Pornhub.com web pages such as page layout, video order, titles and views, authors, categories, and more.
This data collection helped us to determine potential recurring patterns, especially regarding the underlying logics governing the different sections of the homepage.
Observations
- Homepage: it keeps changing even for different users
- Recommended: the personalization based on what the bot saw
- Videos: some of them where removed
F I N D I N G S
* * *
A small summary and next steps
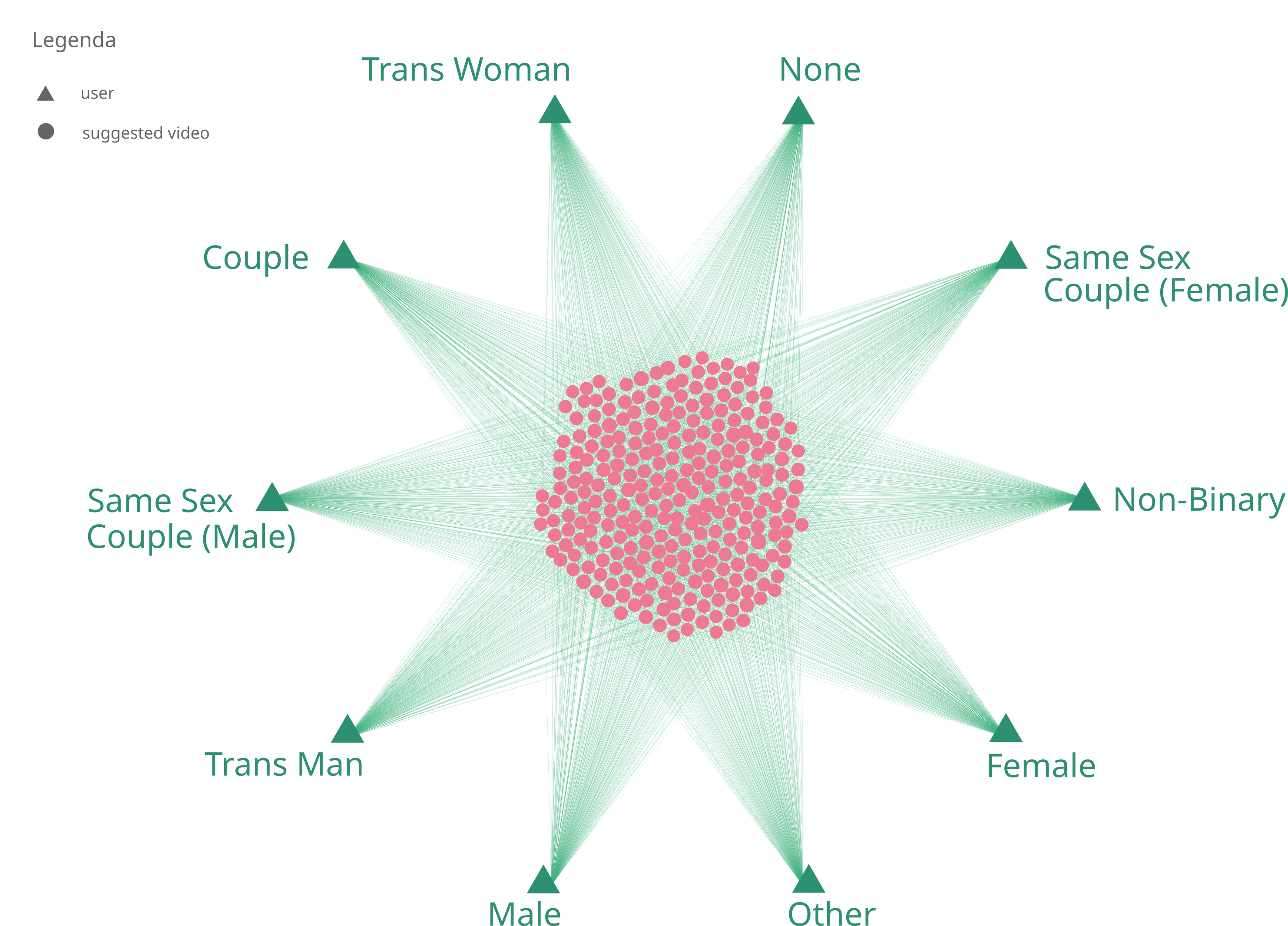
homepages layout
* * *
COMMON SECTIONS
The homepage is not completely individually personalized.
The majority of the sections propose the same videos to all users.
This is the case for:
· Hot Porn Videos in Your Country
· Most Viewed Videos in Your Country
· Recently Featured XXX Videos
personalized content
* * *
RECOMMENDED CATEGORY FOR YOU
Not all 10 profiles shared the same 5 sections
The cluster seem to reflect gender-normativity. This is especially relevant considering that this specific section is missing for Same Sex Couple (female), Non-Binary, Trans Female, and Trans Male.
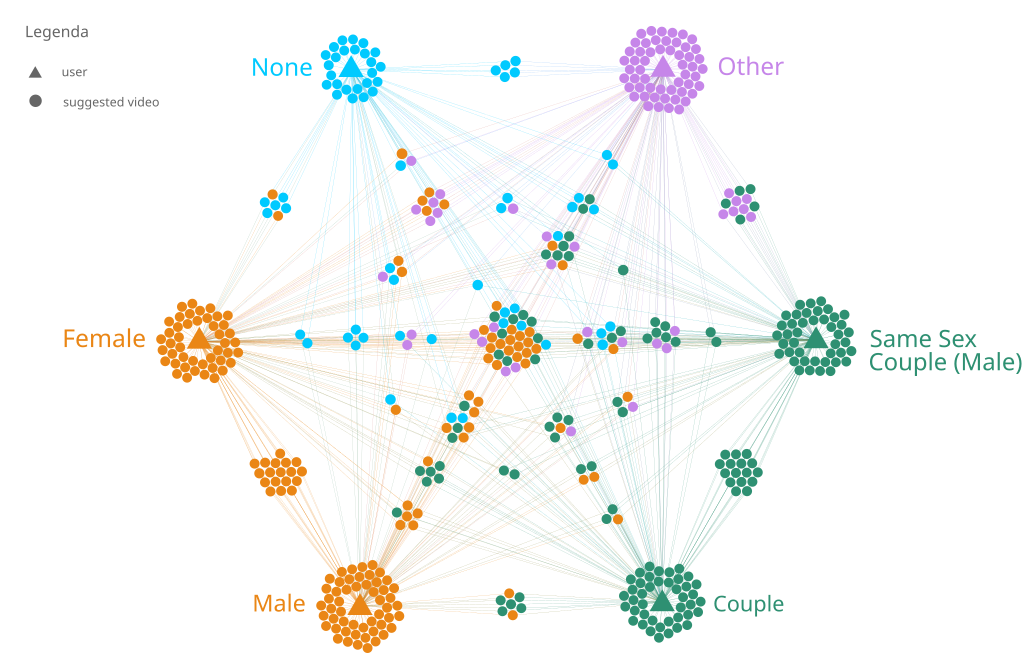
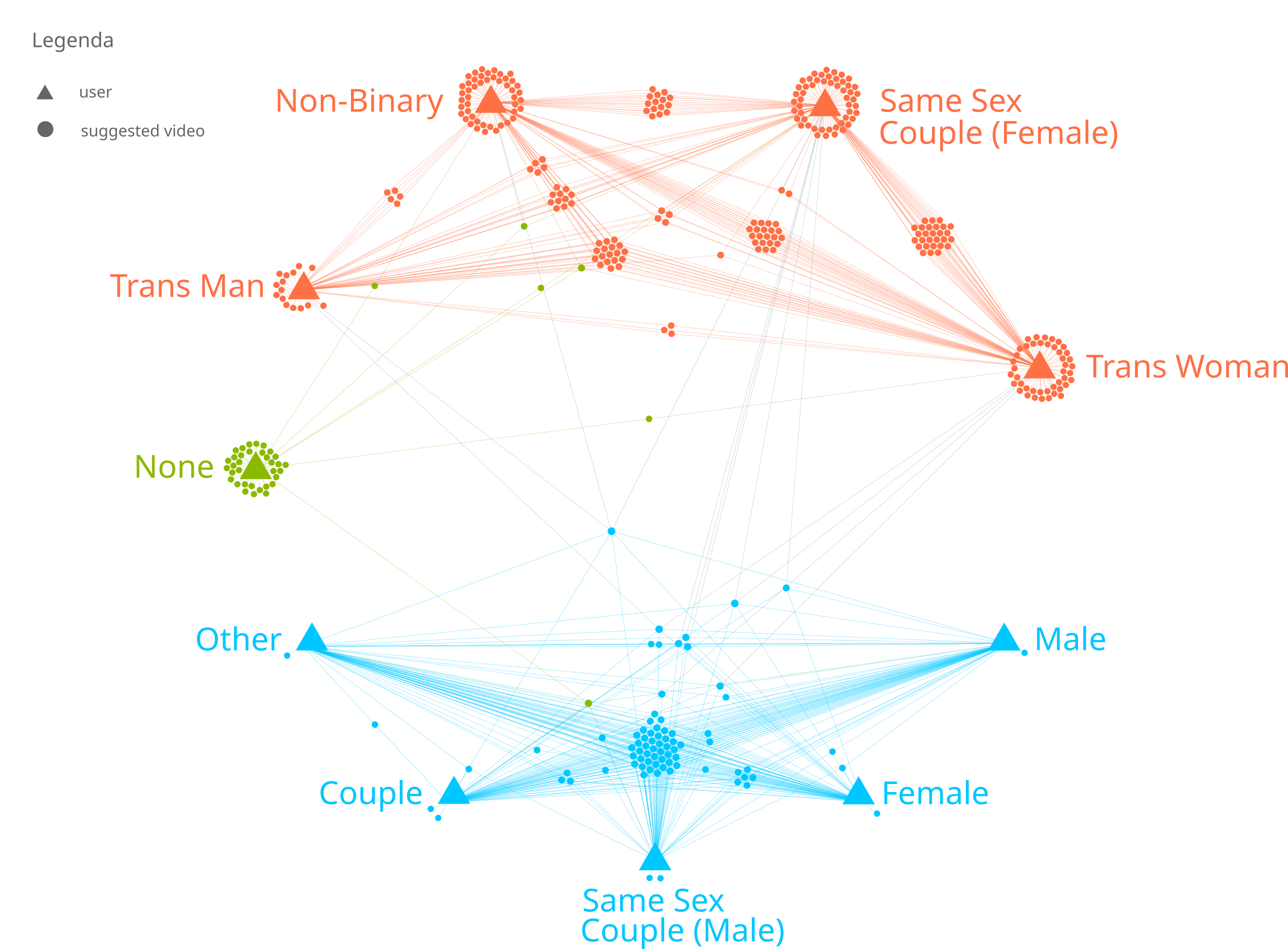
personalized content
* * *
RECOMMENDED
FOR YOU
Common for all profiles
the gender-normative group showcases videos from model, channels, and pornstar; the second group instead does not include channels (production companies). Pornhub manages content in relation to gender identity factoring in broader productive and distributive logics as well?
F U T U R E
D I R E C T I O N S
* * *
-
Leaning into more qualitative methodologies might lead to different (and interesting) results
-
Geographic and Cultural Axis. Analyze geographic differences to understand the effects of potential anglocentrism at the ethnic level.
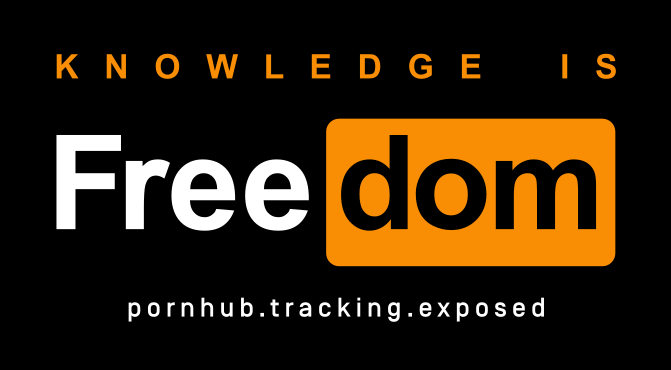
info[@]tracking.exposed
WEtest YOUtube
* * *
A collaborative observation of the Youtube algorithm during the Covid pandemic.
(Academic publication)
(Call to action)
(Analysis notes)
Methodology
March 25th 2020 we openly asked to:
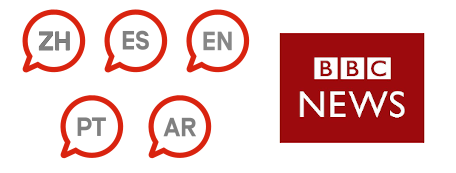
Watch five BBC videos about Covid-19 on Youtube.
In five different languages.
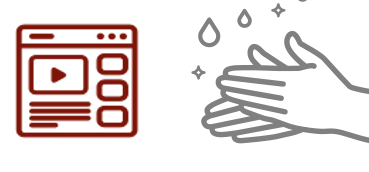
All togheter, compare the algorithm suggestion.
And learn how to wash hands.
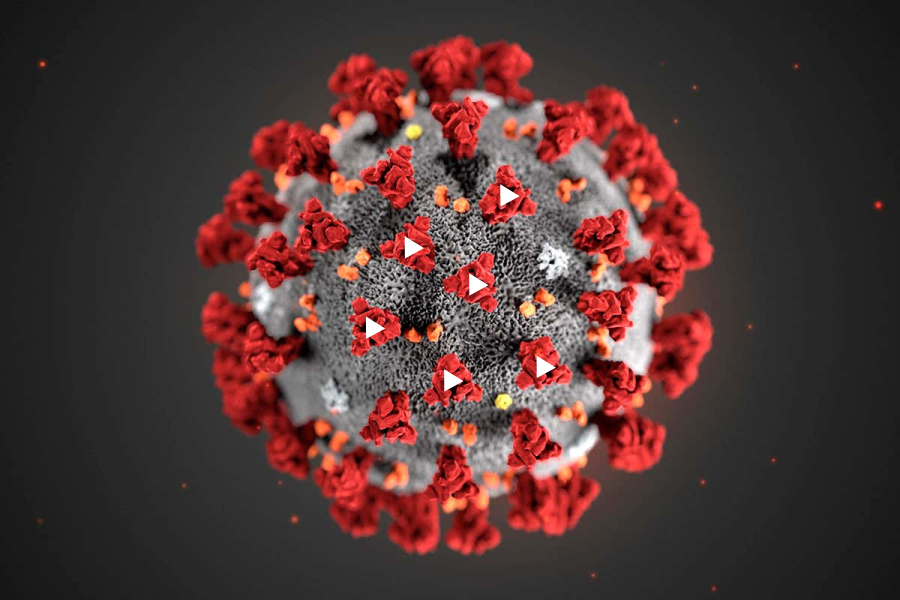
What we observe:
- Recommended videos: Where the personalization algorithm takes action
- Participants comparison: Personalization can only be understood by comparing different users
- Content moderation: What about disinformation? Is there a worst curation on non-english lenguages?
ANONYMIZATION PROCESS
-
01. Unique and secret token
Every participant has a unique code attributed to download his/her evidences
-
02. Your choice
With the token, participants can manage the data provided: visualize, download or delete
-
03. Not our customer
We are not obsessed by you ;) We don't collect any data about your location, friends or similar
-
04. WEstudy YOUtube
We collect evidence about the algorithm's suggestions, like recommended videos
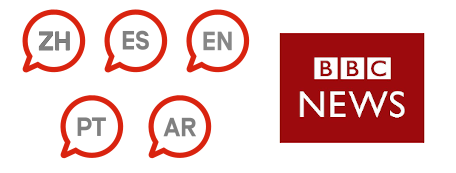
Research Protocol
We asked participants to open, for at least 10 seconds,
a sequence of 5 different BBC videos about Covid-19.
- Open YouTube Homepage
- Open the Chinese video.
- Open the Spanish video.
- Open the English video.
- Open the Portuguese video.
- Open the Arabic video.
- Open again YouTube Homepage
F I N D I N G S
* * *
A small summary of the most interesting results
Distribution of Recommendations
* * *
The vast majority of videos are recommended very few times (1-3 times)
-
-> Summing up, 57% of the recommended videos have been recommended only once (to a single partecipant). -
-> Only around 17% of the videos have been recommended more than 5 times (out of 68 partecipants).
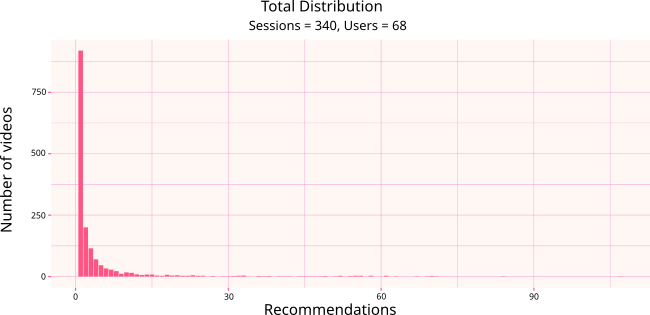
For example, the first bar represents the videos recommended once. They are more than 800.
Distribution of Recommendations
* * *
Analyzing the recommendations of each signle video, the disctibution doesn't change.
-
-> Here you can find the distribution graphs for each lenguage. -
-> The only video suggested to all the participants is a live-streamed by BBC in Arabic. It appears as a recommendation watching the Arabic video.
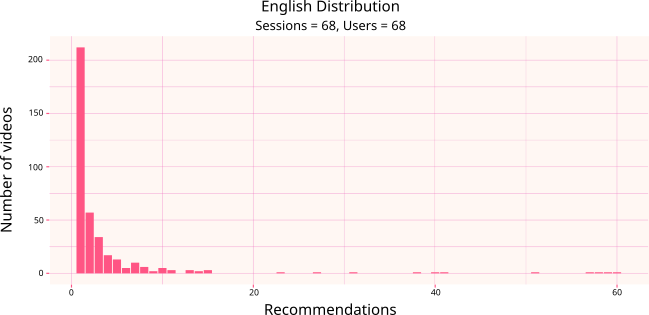
For example, the first bar represents the videos recommended once. They are more than 200.
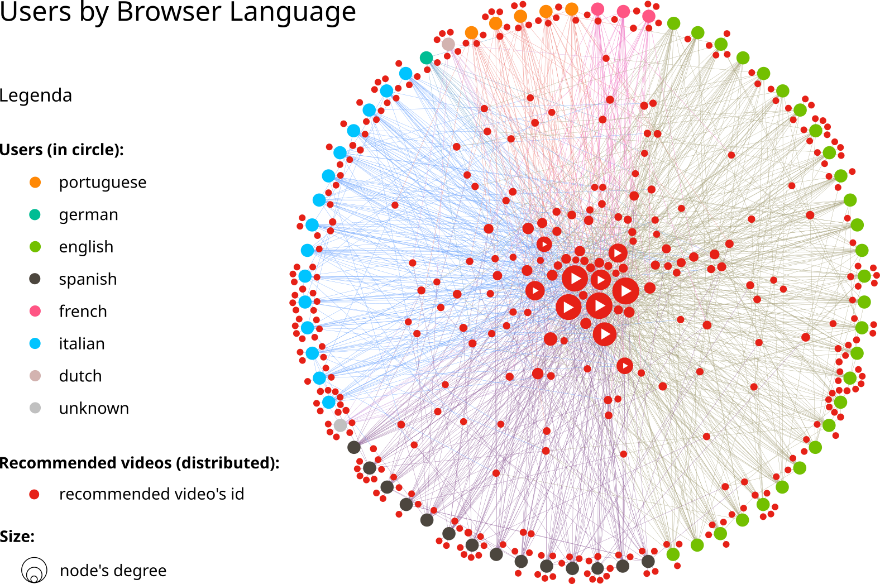
Users in a circle watching the same videos and get really differentiated suggestions (red nodes)
Recommendations Network
* * *
Here we can see the network of recommended videos generated by the Youtube algorithm, comparing the participants.
-
-> Graph of the videos suggested to the participants while watching the video “How do I know if I have coronavirus? - BBC News” -
-> The nodes outside the users in cyrcle are videos suggested to just one participant, they are the most personalized.
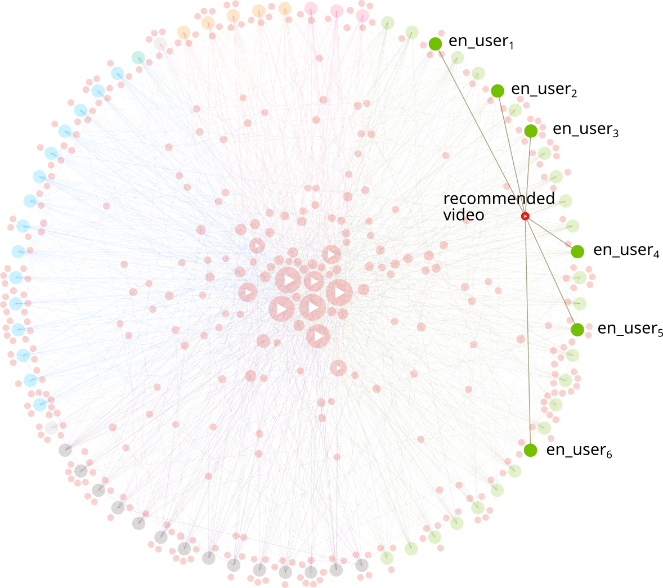
Same graph as the previous slide, with some nodes highlighted.
Recommendations Network
* * *
An example of video suggested just to english-browser participants
-
-> A basic example of how our personal information (as the language we speak) is used to personalize our experience -
-> Here we have a pice of the filter bubble: the algorithm devides us from other users usign our personal information
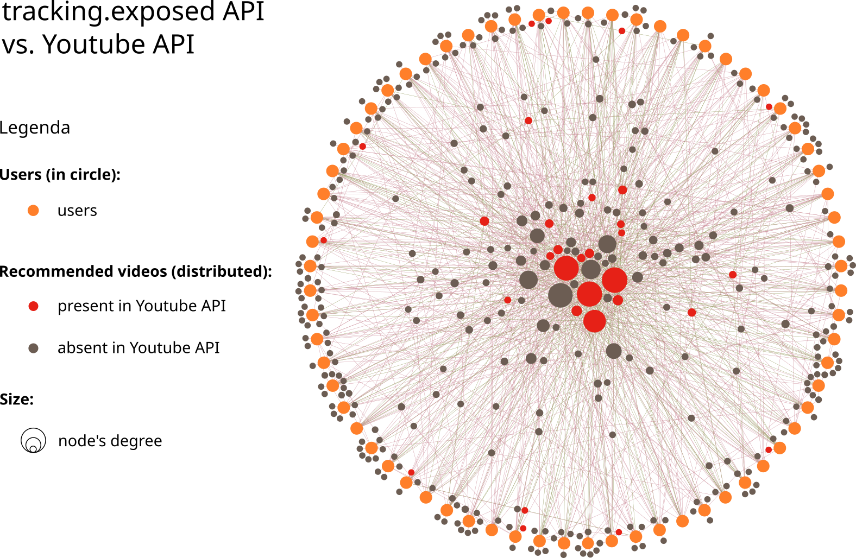
The same graph as before, but the videos that Youtube says should be recommended are red.
Official API? No thanks!
* * *
A comparison between offical Youtube data (OfficialAPI) and our independently collected dataset
-
-> Youtube data are not a good starting point to analyze...the Youtube algorithm! -
-> That's why passive scraping tools like youtube.tracking.exposed are a good way to analyze the platform independently!
Take Out Message
* * *
-
Filter bubbles do exist, and we can measure them
-
Never trust offical API for indipendent research