Algorithmic bias on YouTube during Covid and the US election
-
-
CPDP, Bruxelles, 23 May 2022
Claudio Agosti, @_vecna
-
Who am I? Claudio Agosti
1999 - 2011
Computer security, privacy activism.
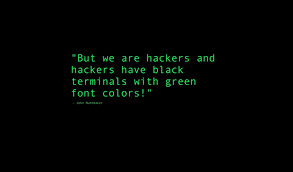
2011 - 2016
NGOs, whistleblowing protection.
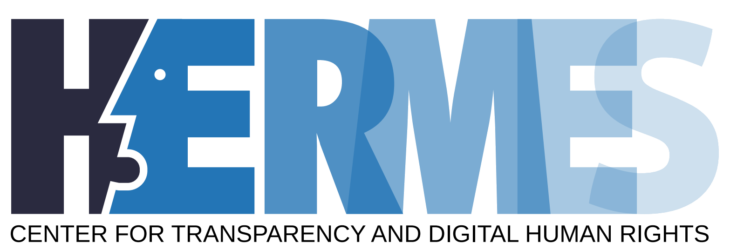


2016+
Founded Tracking Exposed, Research associate.
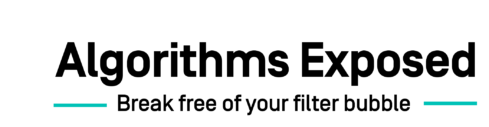
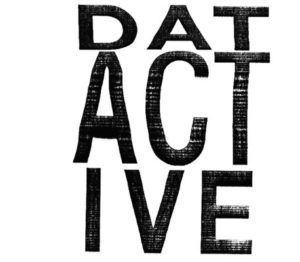
Special trivia
Engineered recipe for the fluffiest pancake
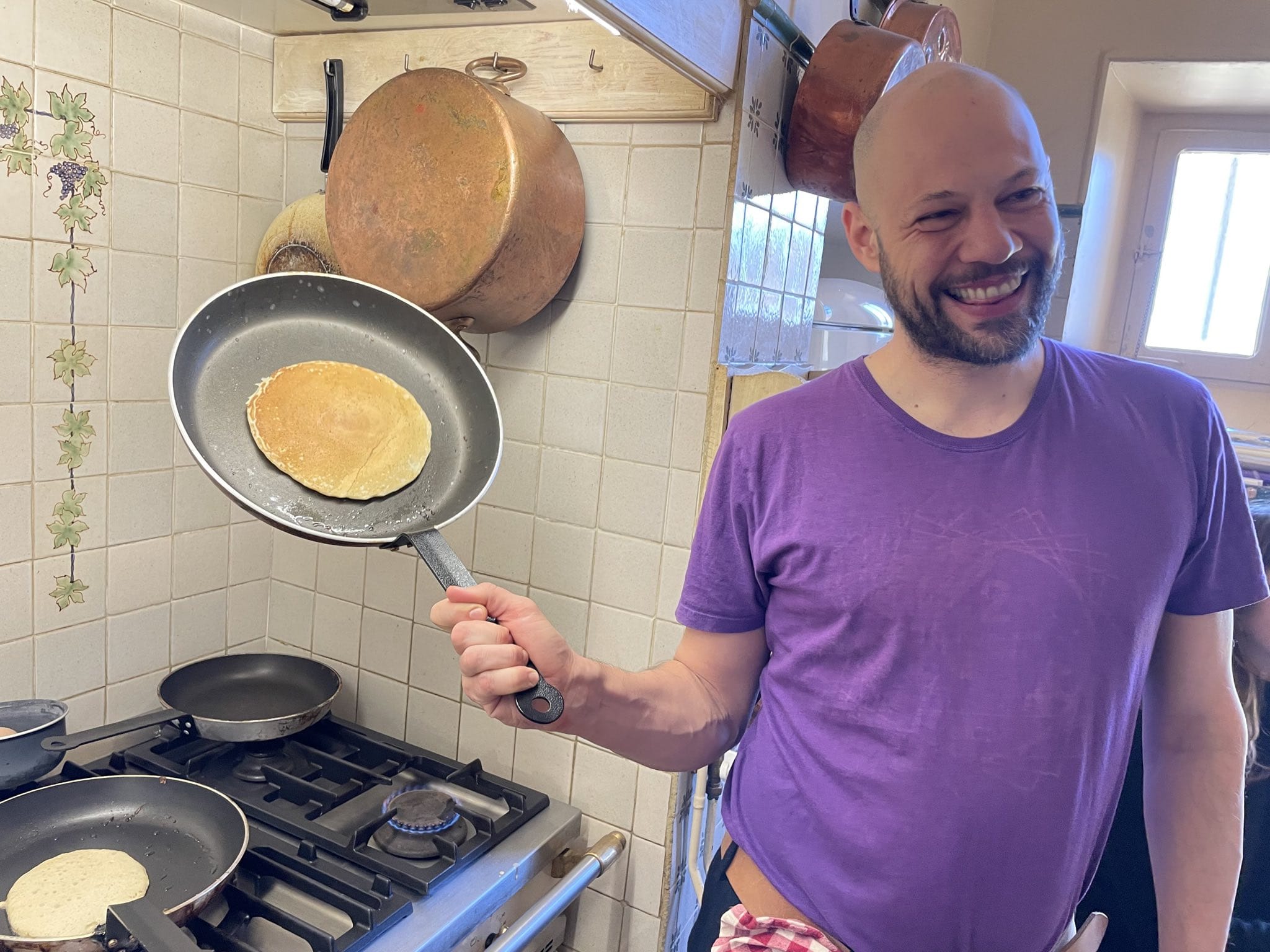
Tracking Exposed is a no-profit organization that produces free software
Currently supported by
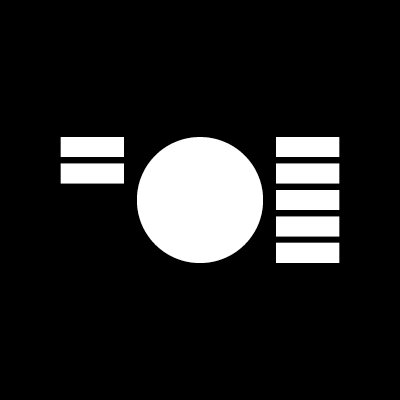
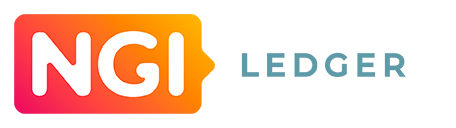
In the past
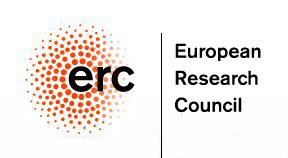
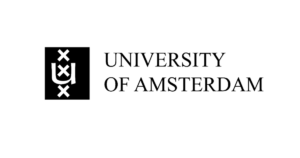
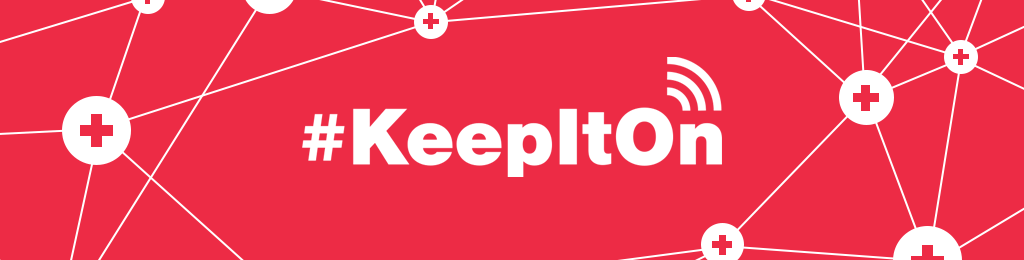
Offering services of
- Third-party algorithm assessment
- Election monitoring
- Workshops and trainings on algorithm analysis
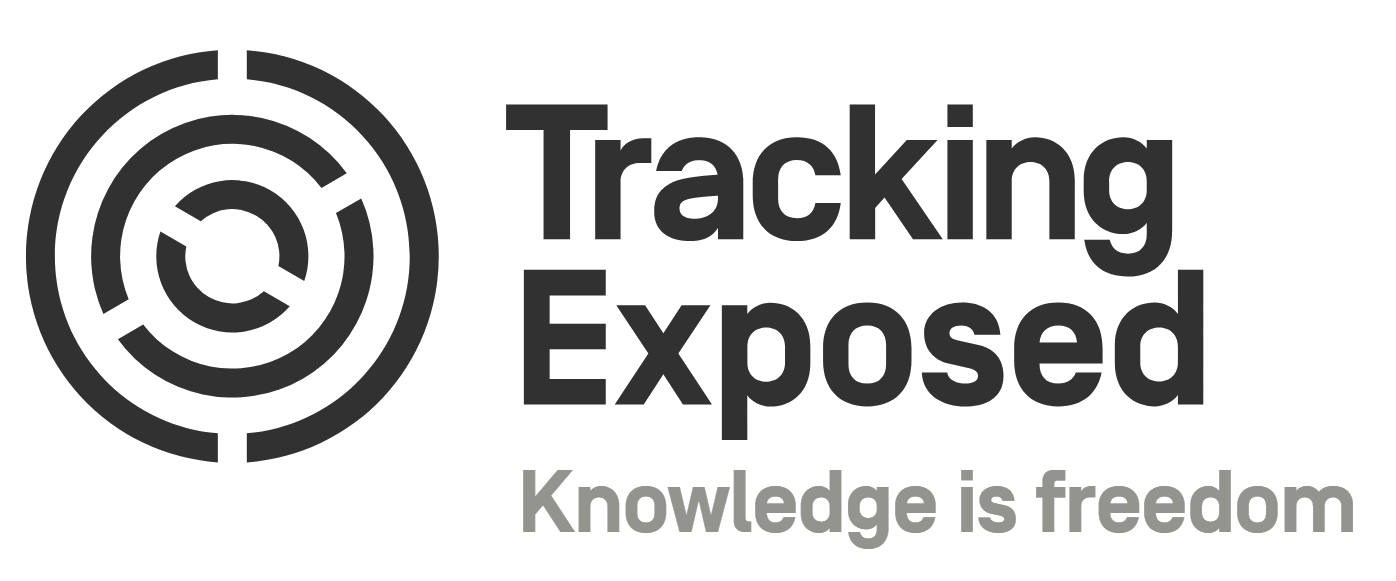
We investigate influential algorithms
Investigation — litigation — free Software — Training
OUR WORK
in development
Narrative example:
Climate Misinformation
* * *
"For the search term “global warming,” 16% of the top 100 related videos included under the up-next feature and suggestions bar had misinformation about climate change."
"YouTube is driving its users to climate misinformation and the world’s most trusted brands are paying for it."
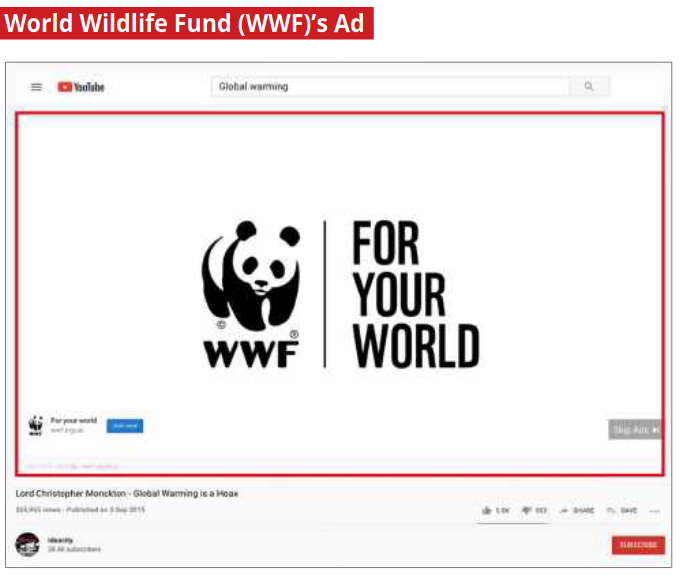
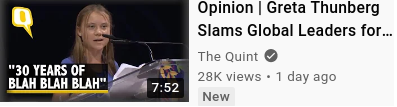
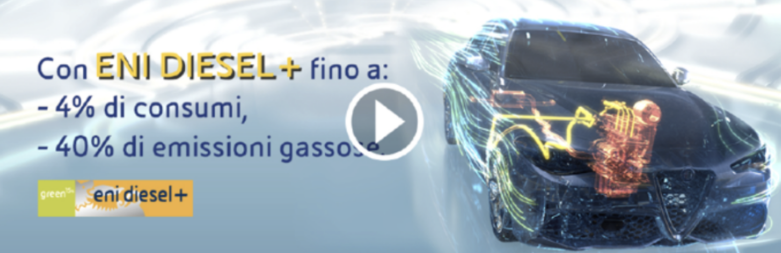
Eni was fined for €5 millions because of "deceptive" claims that palm oil biodiesel is "green", misleading consumers in an advertisement campaign (Wall Street Journal)
Is Greenwashing targeting climate activists?
* * *
Research question
Let's compare the ads provided us while watching climate videos
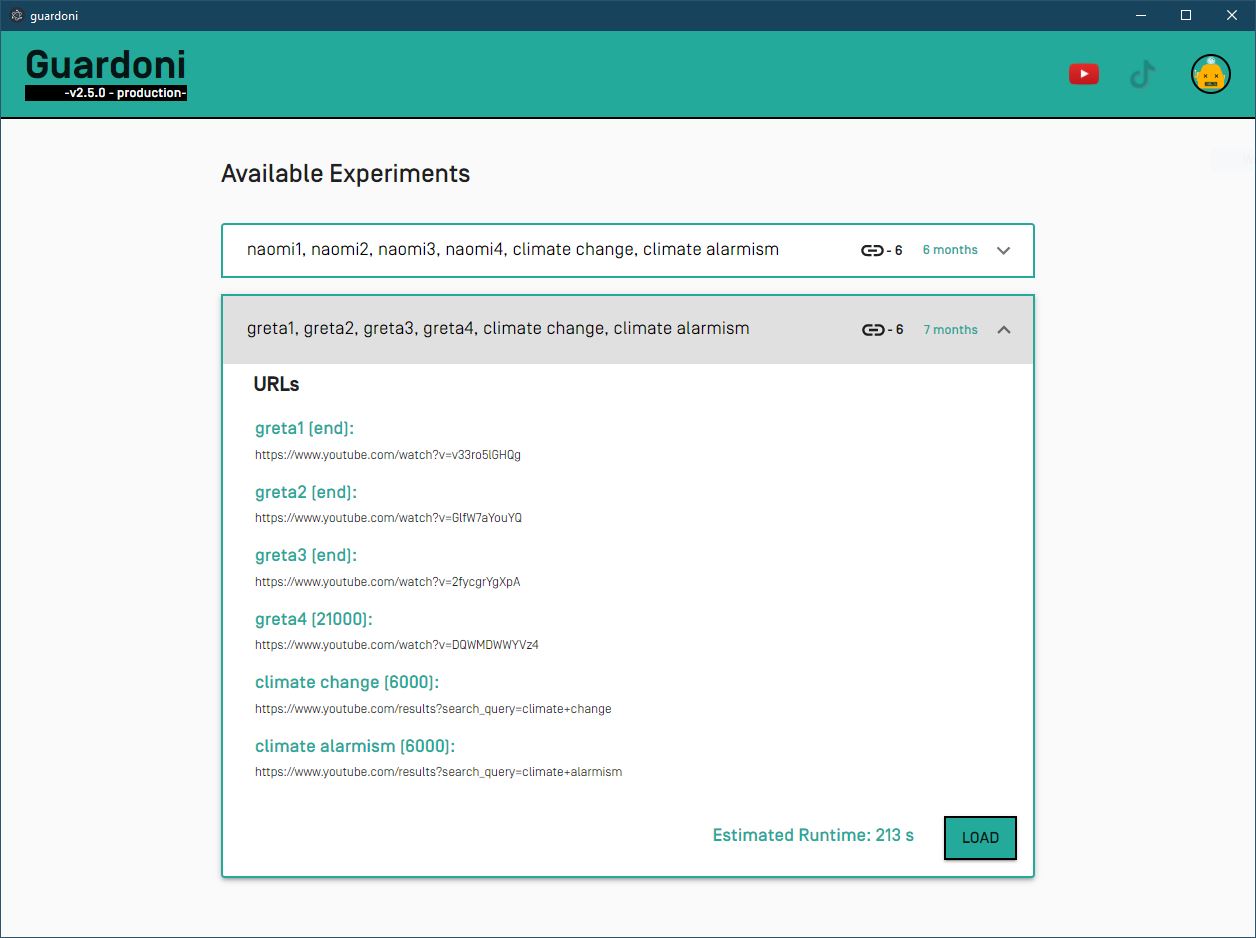
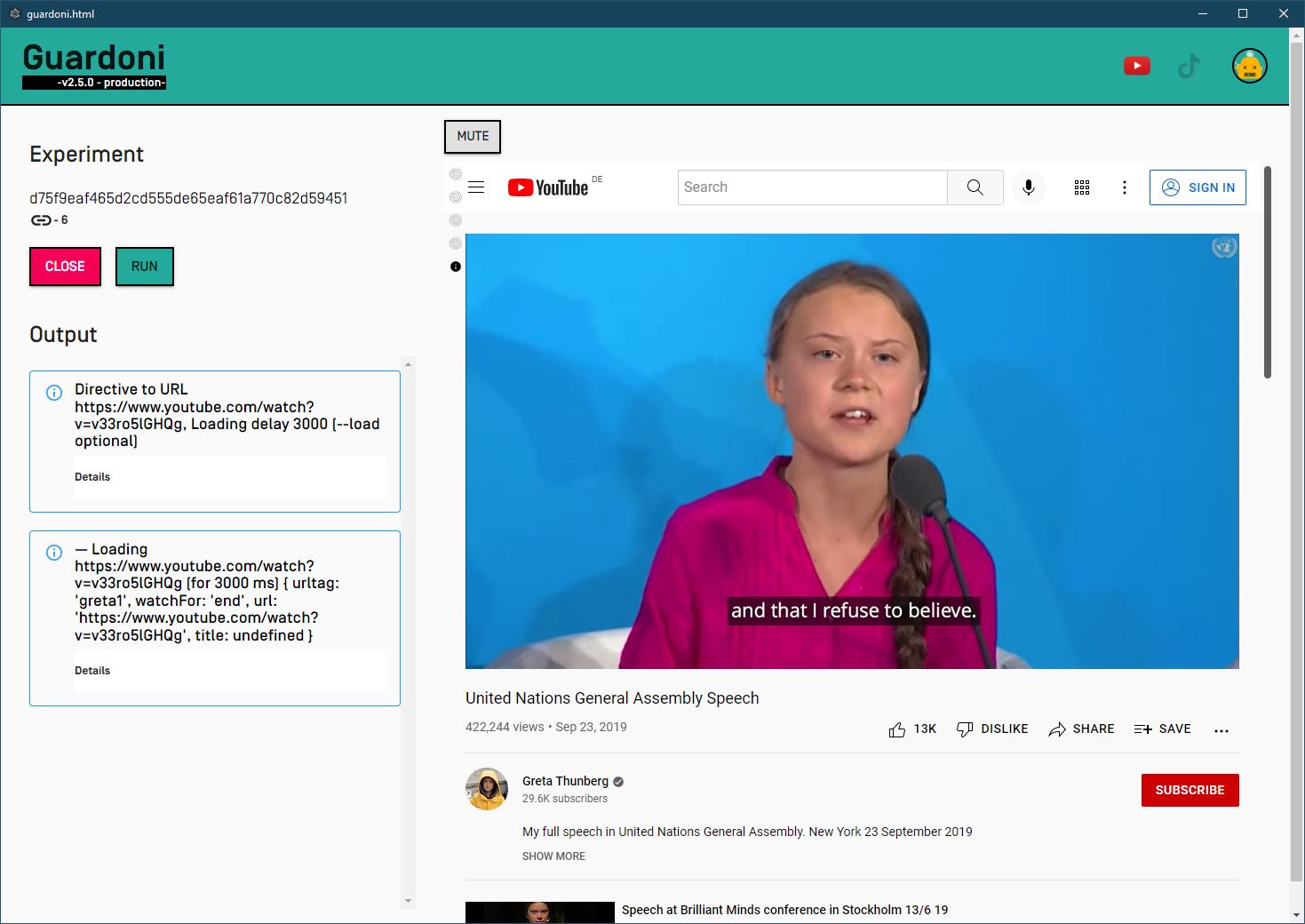
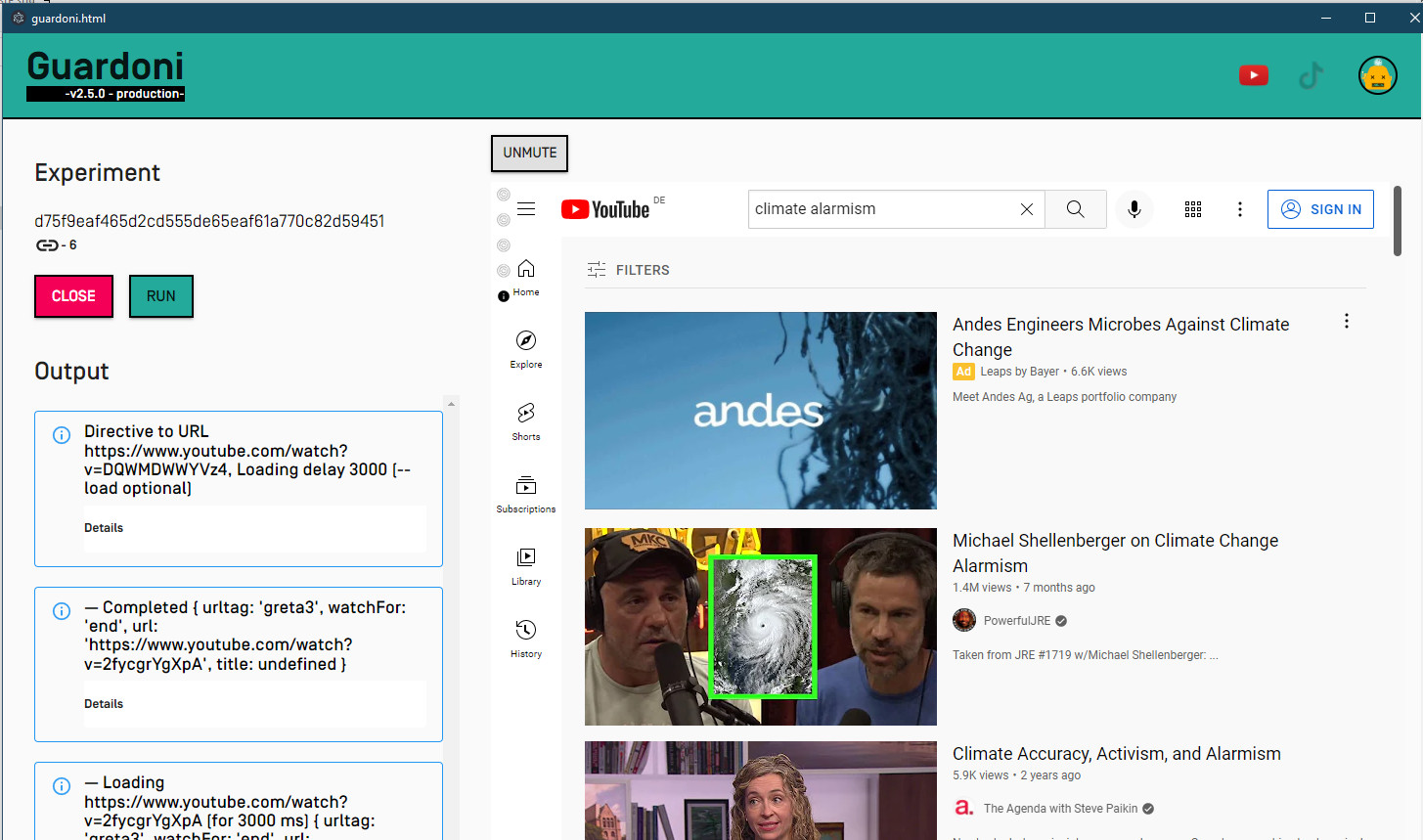
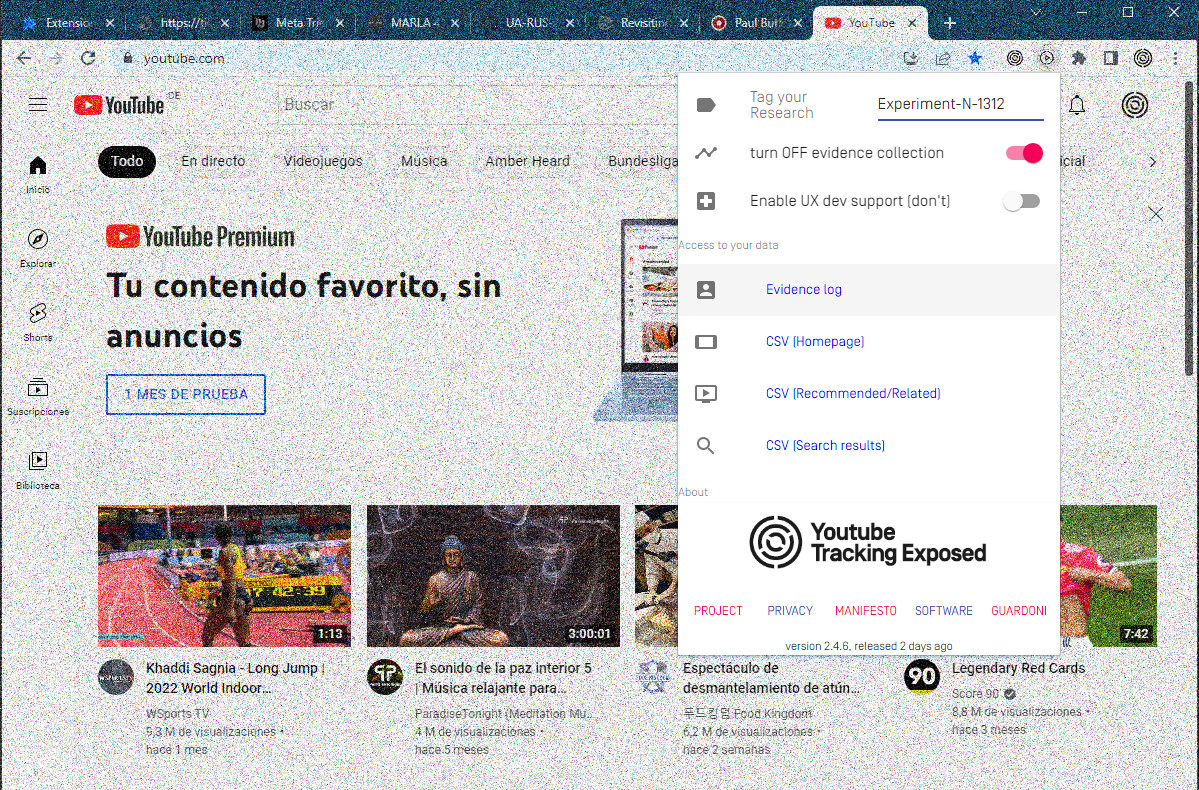
Scraping is a complex business — the supported pages
-
Homepage
Has sections
one kind of advertisment -
Search results
Has sections
one kind of advertisment -
Video page
Has recommendations, 20 default, up to 120
four kind of advertisment
All of these display personalization based on individual, collective, and geographical profiling
THE DATA FORMAT
* * *
JSON|CSV simplified structure
Each entry represent a recommended video from Youtube.
A few are topic-related, a few personalized, and other a mix of the two.
{ "savingTime": "2021-08-31T17:27:06.213Z", "watcher": "muffin-rhubarb-cheese", "blang": "en-US", "recommendedVideoId": "hFISmpbEg1g", "recommendedPubtime": "2018-08-31T17:50:59.000Z", "recommendedForYou": "YES", "recommendedTitle": "What A Difference A Day Made", "recommendedAuthor": "Jamie Cullum", "recommendedVerified": true, "recommendedViews": 3737849, "watchedId": "q-lPwo1GUKw", "watchedAuthor": "Jamie Cullum", "watchedTitle": "But For Now", "watchedViews": 3572985, "watchedId": "q-lPwo1GUKw", },
How personalization looks like?
The students watch the same video, and record their personalization, so we compare how youtube has recommended videos to them.
you're watching a condition of reduced personalization.
Each black dot is a student.
Each violet bubble in the center represents one of the video suggested.
We tried to reduce these differences, to have something similar to a *non-personalized* algorithm stage.
Same room, same studends, same day, same computers, but logged browsers
It is visually clear how the data points linked to the profiles cause personalized suggestions.
🦠...and then COVID-19🦠
Can YouTube Quiet Its Conspiracy Theorists?
— via New York Times
2nd of March 2020
Youtube spokeperson Farshad Shadloo said
the company was continually improving the algorithm that generates the recommendations. “Over the past year alone, we’ve launched over 30 different changes to reduce recommendations of borderline content and harmful misinformation, including climate change misinformation and other types of conspiracy videos,” he said. “Thanks to this change, watchtime this type of content gets from recommendations has dropped by over 70 percent in the U.S.”March 25th 2020 we openly asked to
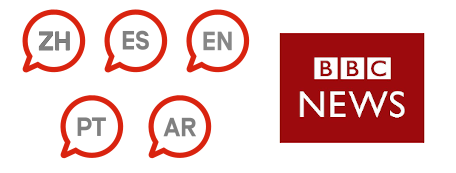
Watch five BBC videos about Covid-19 on Youtube.
In five different languages.
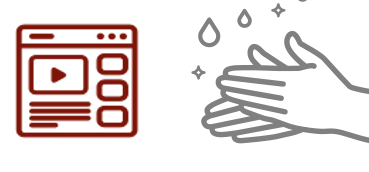
All togheter, compare the algorithm suggestion.
And learn how to wash hands.
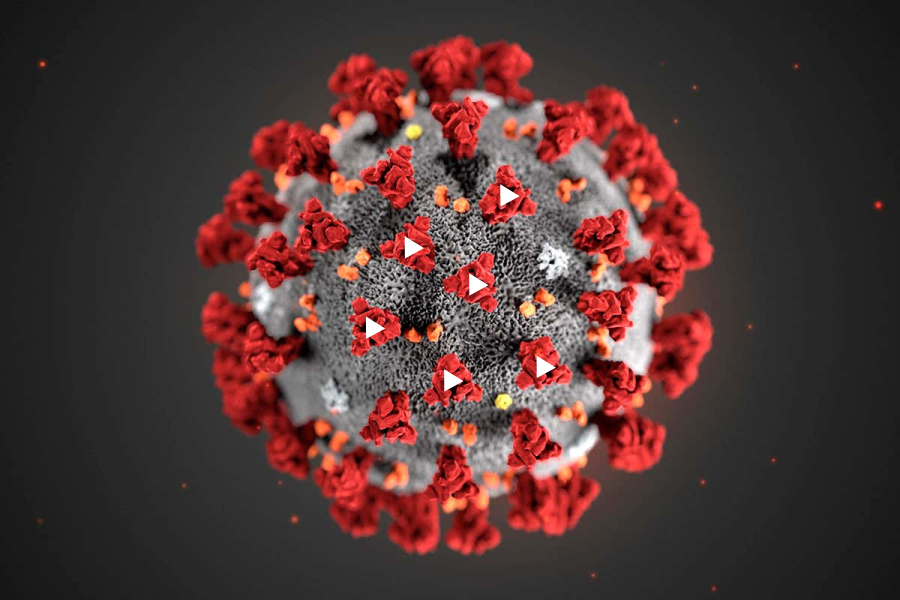
What we observe:
- Recommended videos: Where the personalization algorithm takes action
- Participants comparison: Personalization can only be understood by comparing different users
- Content moderation: What about disinformation? Is there a worst curation on non-english lenguages?
ANONYMIZATION PROCESS
-
01. Unique and secret token
Every participant has a unique code attributed to download his/her evidences
-
02. Your choice
With the token, participants can manage the data provided: visualize, download or delete
-
03. Not our customer
We are not obsessed by you ;) We don't collect any data about your location, friends or similar
-
04. we study youtube
We collect evidence about the algorithm's suggestions, like recommended videos
F I N D I N G S
* * *
A small summary of the most interesting results
Distribution of Recommendations
* * *
The vast majority of videos are recommended very few times (1-3 times)
-
💡 Summing up, 57% of the recommended videos have been recommended only once (to a single partecipant). -
💡 Only around 17% of the videos have been recommended more than 5 times (out of 68 partecipants).
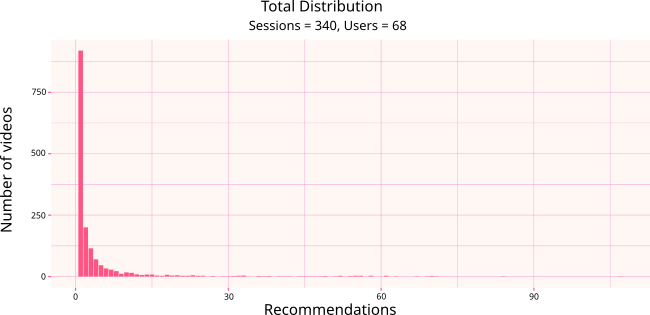
For example, the first bar represents the videos recommended once. They are more than 800.
Distribution of Recommendations
* * *
Analyzing the recommendations of each signle video, the disctibution doesn't change.
-
💡 Here you can find the distribution graphs for each lenguage. -
💡 The only video suggested to all the participants is a live-streamed by BBC in Arabic. It appears as a recommendation watching the Arabic video.
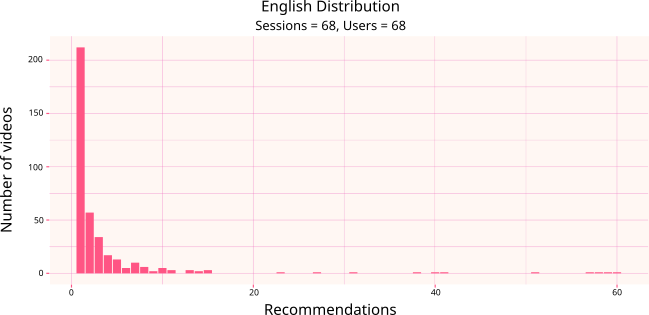
For example, the first bar represents the videos recommended once. They are more than 200.
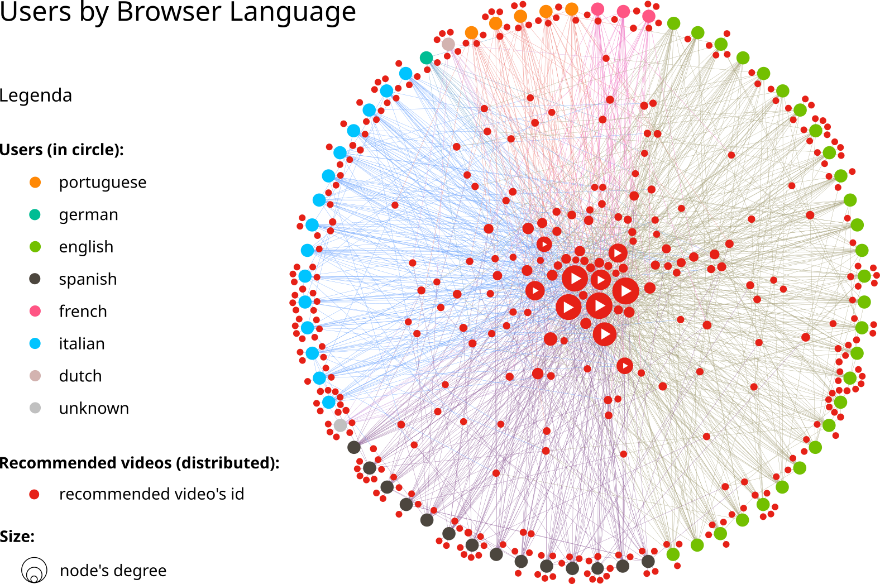
Users in a circle watching the same videos and get really differentiated suggestions (red nodes)
Recommendations Network
* * *
Here we can see the network of recommended videos generated by the Youtube algorithm, comparing the participants.
-
💡 Graph of the videos suggested to the participants while watching the video “How do I know if I have coronavirus? - BBC News” -
💡 The nodes outside the users in cyrcle are videos suggested to just one participant, they are the most personalized.
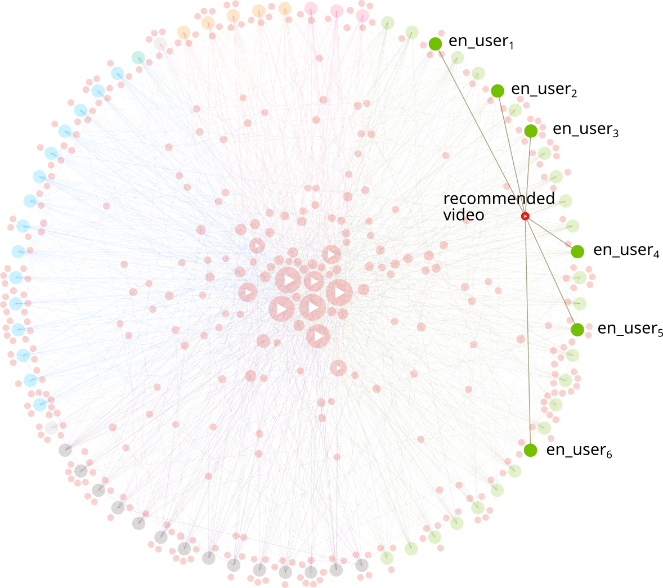
Same graph as the previous slide, with some nodes highlighted.
Recommendations Network
* * *
An example of video suggested just to english-browser participants
-
💡 A basic example of how our personal information (as the language we speak) is used to personalize our experience -
💡 Here we have a pice of the filter bubble: the algorithm devides us from other users usign our personal information
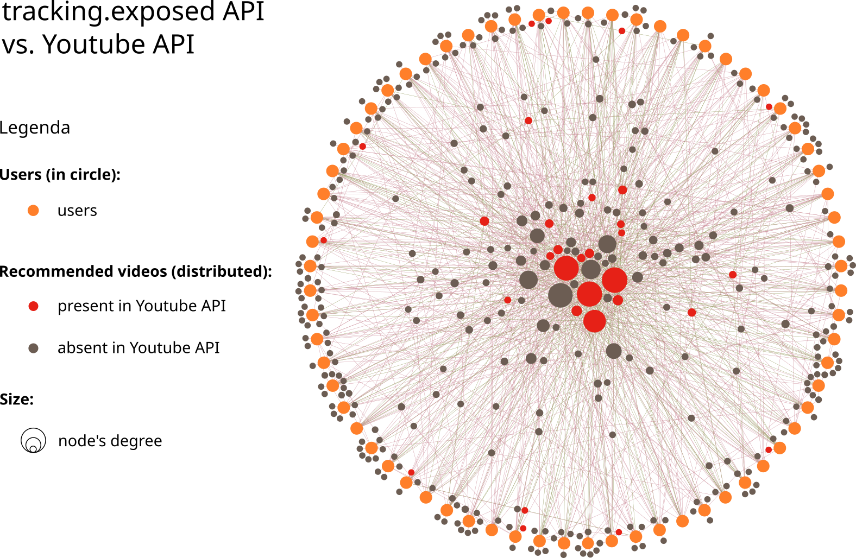
The same graph as before, but the videos that Youtube says should be recommended are red.
Official API? No thanks!
* * *
A comparison between offical Youtube data (OfficialAPI) and our independently collected dataset
-
💡 Youtube data are not a good starting point to analyze...the Youtube algorithm! -
💡 That's why passive scraping tools like youtube.tracking.exposed are a good way to analyze the platform independently!
Takeaways
* * *
💡 Filter bubbles do exist, we propose methods to measure them
💡 Never trust official API for indipendent research
💡 Content moderation is proprotionally effective as much as a country is worthy for Google market
RESEARCH QUESTIONS:
does Youtube personalize the search results?
Can we artificially put a profile under our control, into an echo chamber?
Does YouTube’s algorithm contribute to filter bubbles with their search results?
Which are the qualitative and quantitative differences among echo chambers?
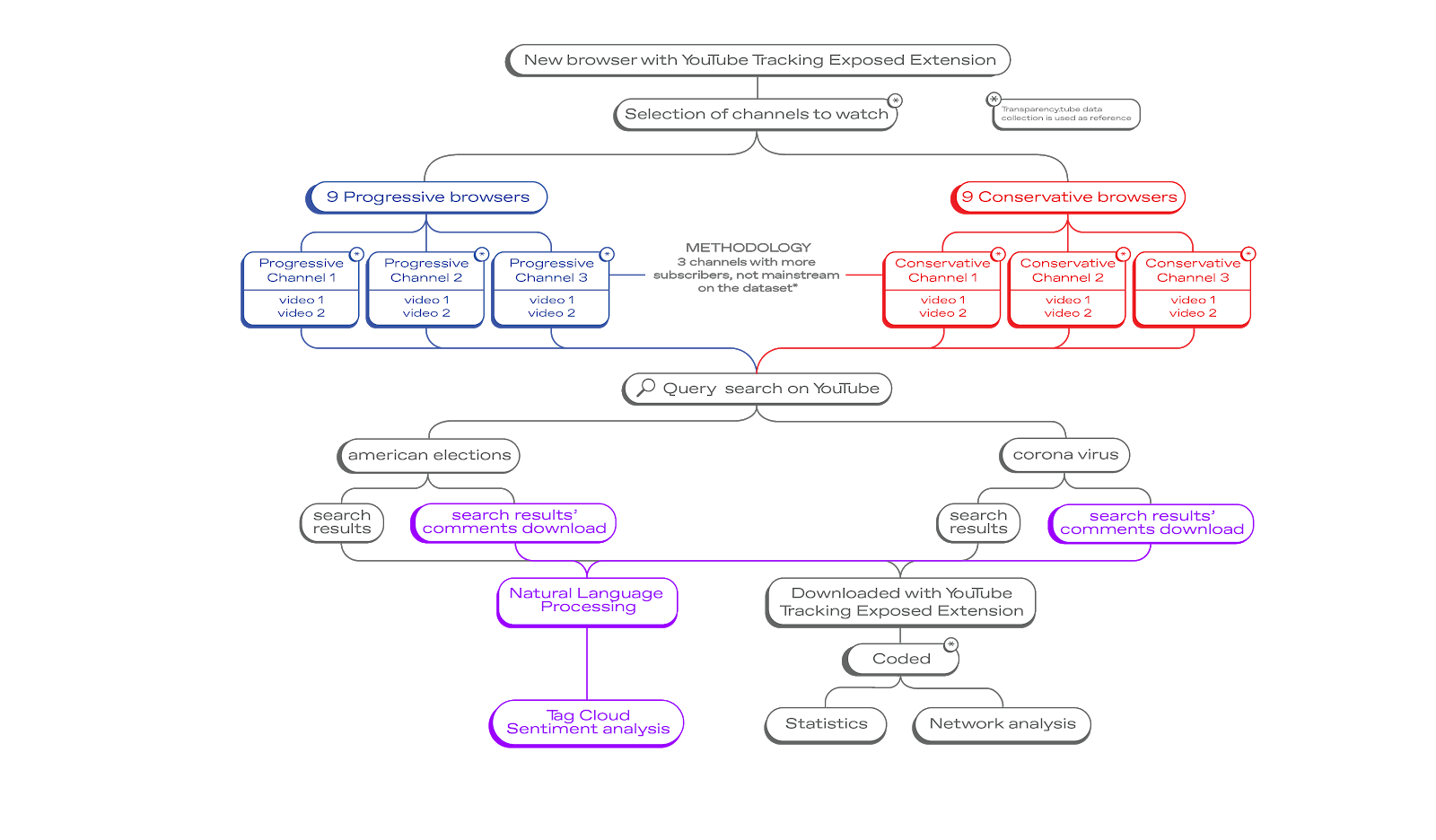
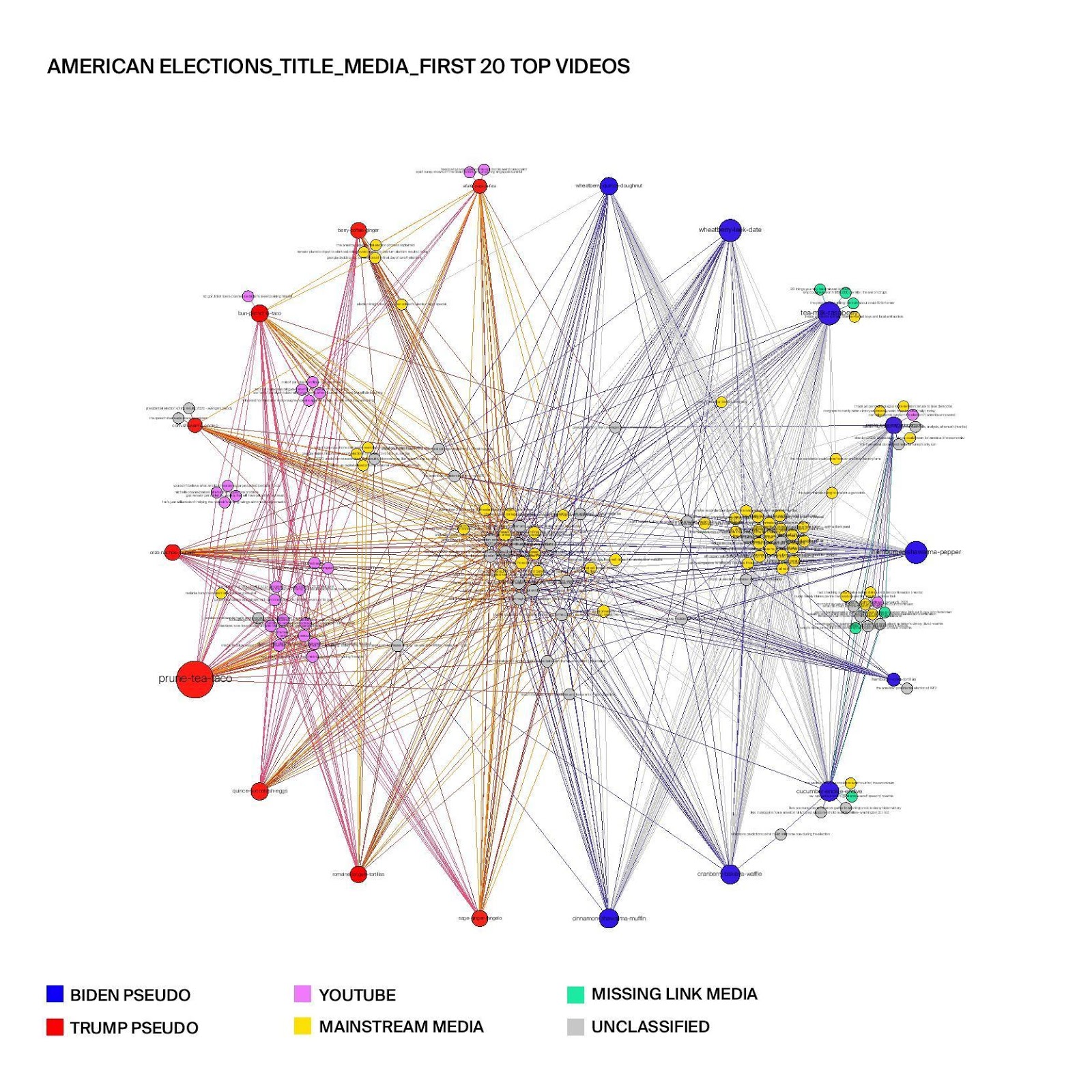
💡 Few activities are enough to profile your search results
💡 Politically oriented videos are enough to personalize a profile
💡 The nature of the media seems to be an emergent pattern tight to political leaning.
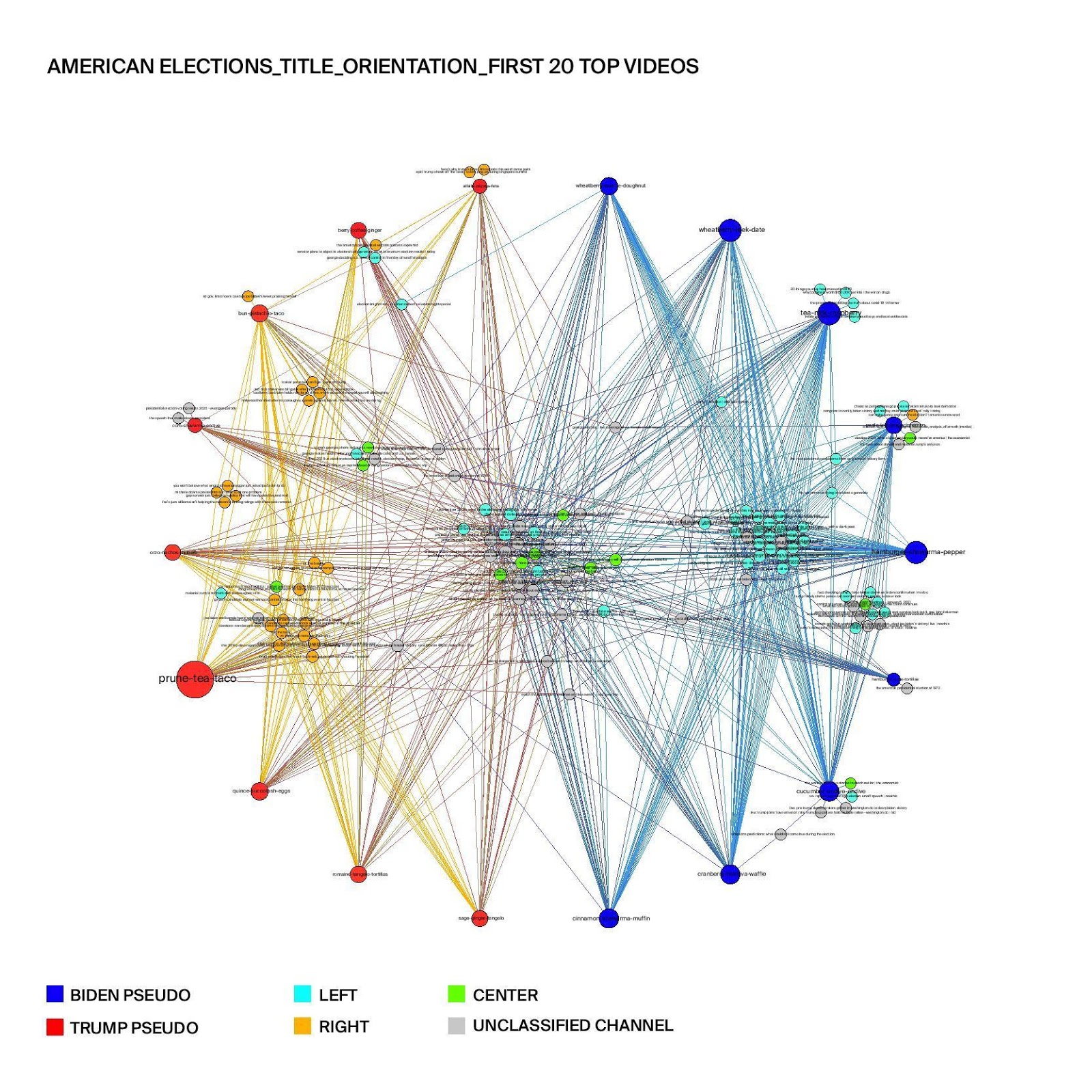
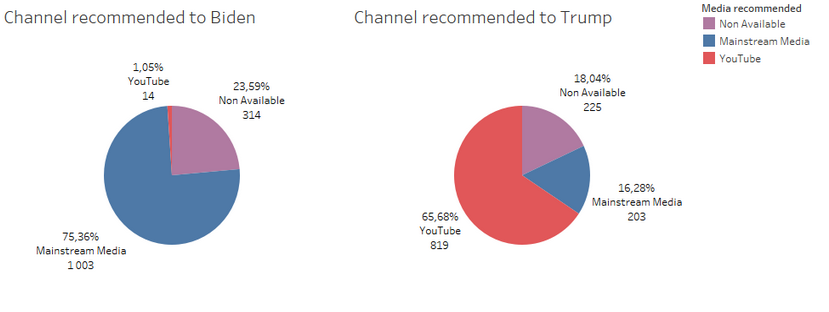